Personalization is at the heart of today’s consumer brand companies. It defines the experience of the brand in the eyes of customers and prospects and drives the underlying economics of the business. And personalization at scale is not just a phenomenon outside the brand; it describes a fundamental shift in how operations happen inside the brand. The value of the brand is measured not just in profit and loss, but in reach and engagement, customer retention and loyalty — driving every action in the business through the lens of customer lifetime value.
In order to fuel transformation, brands need a collection of capabilities forming an engine for customer data that can power their entire enterprise — from analytics to customer support to finance to marketing to compliance. This approach is grounded in technology, but it goes beyond the realm of IT. When implemented successfully it sets the rest of the business up to personalize their efforts and speak to customers as individuals. The customer data engine for any given brand is “every system across the organization that touches customer data” — this broad view grants a holistic look at the ecosystem of connected infrastructure required to deliver the promise of personalization. From talking with leading brands, there are seven common steps to building a successful customer data system.
This diagram shows the seven steps in the middle. Read on for a detailed explanation of each.
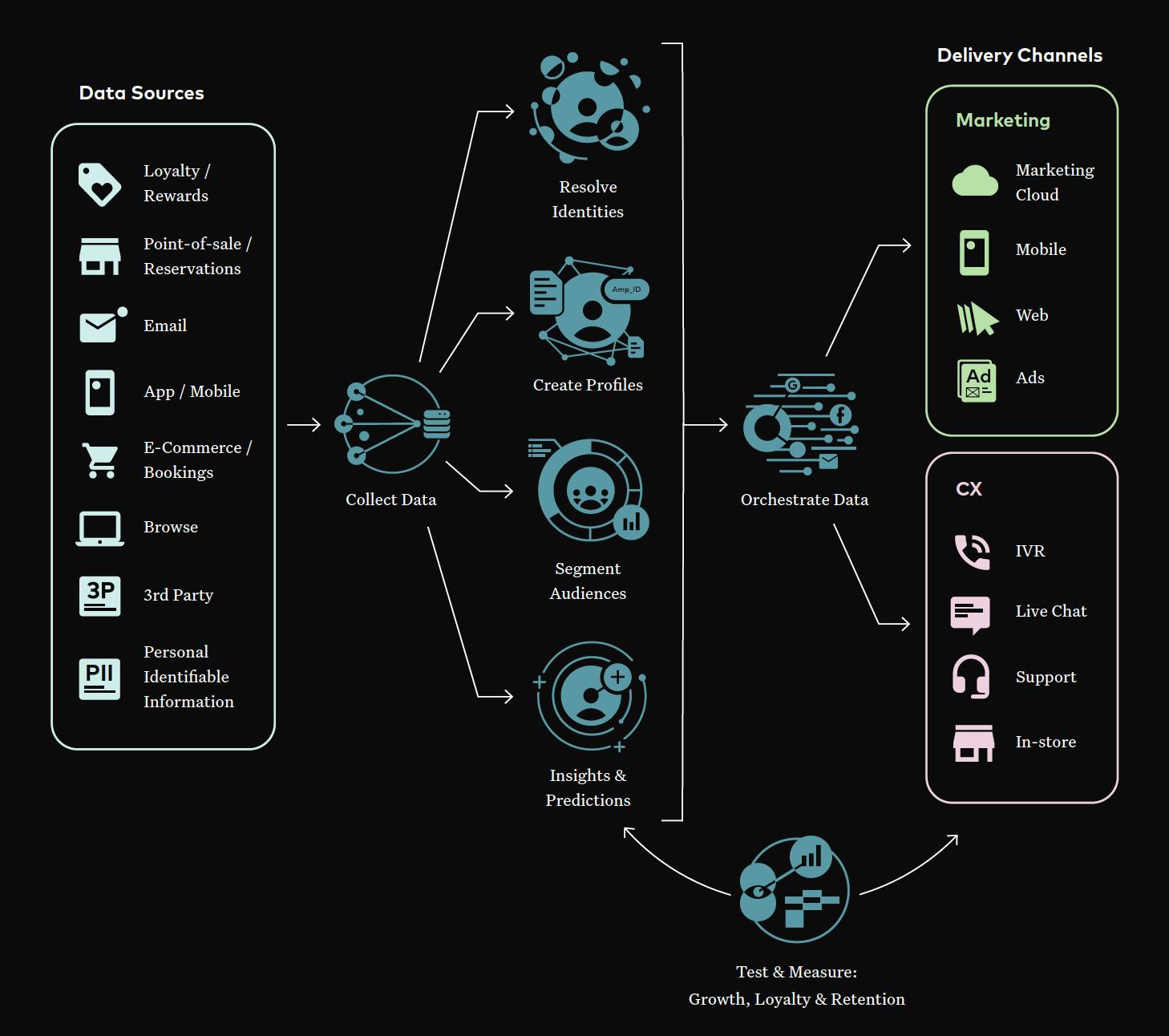
Step 1 - Collect All The Data
The first step in a customer data engine is getting access to all the data about the customer across the brand. This includes all the first-party data available across all channels, online and offline, including the full historical dataset. It may include data licensed from third-party providers or other data collected from resellers or partners. This is the foundation for a complete customer view, and needs to be up-to-date with the latest changes in customer profile information, transactions, behaviors and interactions.
Having this data centrally collected allows for flexibility and iteration that adapts to the changing needs of a business. Typically this requires a broad set of connectors to different systems — including real time streams, API-based connections, and the ability to ingest large volumes of raw historical data. This data should be available in its full form and not require complex ETL (extract-transform-load) to be ingested. This is because, in order to build a complete customer view, it is important to have access to all of the information about customers, not just a sub-set. This collection of data represents the raw ingredients necessary to power your customer data engine.
Step 2 - Resolve Customer Identity
The second step is to take that raw data and identify the building blocks used to understand the customer, the first of which is customer identity. This is the linking key that unlocks the full view of a customer, and it must be refreshed every day so that it’s up-to-date and accurate. This key allows the rest of your customer data to be attached to the right customer — so transactions, visits, support experiences, and interactions can be accurately assigned to the same person. It is critical that this phase include the ability to identify and match customers using only first-party data owned by the brand, particularly given the changes in the third-party data ecosystem driven by increased privacy regulation and changes in how browsers and phones manage cookies and mobile advertising IDs.
The identity resolution step is foundational to everything that happens downstream — if identity is inaccurate or not up-to-date it means that every other calculation and action will suffer from an incomplete view of the customer.
Step 3 - Build the Customer Profile
Once the data is linked together, the next step is to build a complete customer profile. This profile should provide the single view of the customer that powers all parts of the brand — from analytics to customer support to marketing to finance. Because the business changes all the time, customer data changes as well — which means that flexibility is a must. It should be easy to add new attributes to the profile, add in new data sources about the customer, and create brand- or role-specific views of the information that meet the changing needs of the brand.
Change management and change control is crucial, including the ability to validate changes before they are used in production, since changes can have a material impact on the quality of analytics and marketing campaigns that power the business. Self-service capability is also critical, as the brand needs to be able to respond quickly and cannot be locked into a fixed view.
Step 4 - Generate Insights & Predictions
Next it’s time to put the data to work. This capability is made up of two key pieces: what insights you derive from customer data at face value and what machine learning and AI can predict about customer activity based on historical data. Insights should be generated at the customer and segment-level, including brand and channel behaviors, product preferences, revenue sizing, and recommended actions.
Because real-time personalization is a business driver, it’s also important to visualize and monitor customer-centric metrics and KPIs that highlight shifts in customer economic indicators, identifying risks and opportunities. Additionally, predictive models can help marketers identify which segments, personas, or audiences have the highest growth opportunity, affinity for specific products, or churn propensity, all of which can be applied to improve campaign ROI and customer lifetime value.
If identity is inaccurate or not up-to-date it means that every other calculation and action will suffer from an incomplete view of the customer.
Step 5 - Audience Segmentation
The fifth step is to organize the profile data and segment into different audiences for analytics and marketing. Common audiences include first-time buyers, high-value customers, customers who are at risk of churning, or members of the loyalty program. The right historical and predictive attributes are a must to drive segmentation — examples include up-to-date historical and predictive lifetime value, preferred product or preferred channel, and an up-to-date view of contactability, including opt-in status.
It is important that this segmentation capability allow for rapid exploration that can be used for measurement (growth in loyalty signups), analytics (impact of campaign activity), and marketing (opportunity sizing). Segmentation needs to scale to serve sophisticated SQL users as well as marketing and business users, powering both analytics and marketing. Any segmentation approach must fit in with the data infrastructure of the brand — including rich connections to marketing clouds and data warehouse platforms.
Step 6 - Orchestration & Distribution
The sixth step is to distribute (or orchestrate) the customer data to all of the downstream systems used across the brand for analysis, engagement, business operations, and insight. Companies have invested years in training marketers and analysts on world-class tools — from data visualization to campaign management to customer experience — and there is no need to replace these systems. Instead, a customer data engine powers them with better data. The engine needs to power the full distribution of the customer master, as well as lists and up-to-date attributes, across all systems in the brand.
Step 7 - Measure, Learn, & Iterate
Last, but possibly most important, the combined customer data systems must support rapid measurement, learning, and iteration. This starts with the ingestion of all engagements and interactions in real time to power up-to-date metrics about customer and business health, including the ability to measure the results of campaigns or actions being taken.
This means the engine needs to support a rapid iteration from Step 1 through Step 6. An equally important part is the ability to adapt the systems to changes in the business — new data sources coming online, acquisitions and mergers bringing companies together, and new tools coming to market. A brand’s network of customer data systems can no longer just be resilient to change; it must embrace it.
Putting It All Together
These seven broad steps describe capabilities that are required in any brand ecosystem to drive personalization at scale. And they must fit in with the technology stack of the brand, so business leaders require solutions that meet them where they are. In some cases, brands will want a customer data platform (CDP) that powers all seven steps. In other cases, brands will choose to use a marketing cloud for segmentation and audience creation, while picking a CDP for data collection, identity, and profile creation. And in other cases brands will collect data in a data warehouse and then use a CDP for identity extraction, profile creation, and orchestration throughout their ecosystem. This points to a final consideration when building out the capabilities to power personalization: any tool or vendor should be able to plug seamlessly into the other systems involved.
This is one of the reasons we built Amperity with the flexibility to power any or all of these stages that drive transformation, and is what allows us to stay laser-focused working with business leaders at brands on accelerating transformation that builds from where they are.
Now that you know about the capabilities that power personalization at scale, learn more about putting it into practice and what common pitfalls to avoid in our in-depth guide, This Time It's Personal: The Enterprise Guide to Personalization at Scale.